Looking for a role at a prestigious organization where I can apply my professional talents to improve and expand my organization's business while...
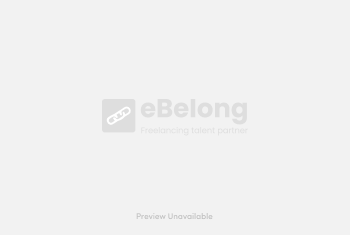
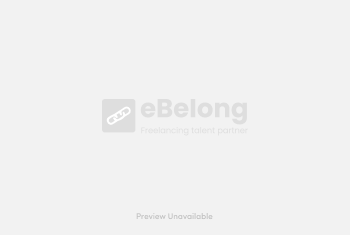
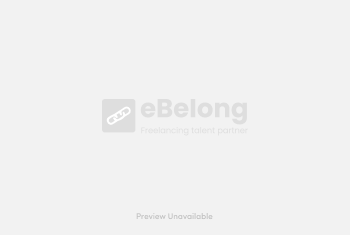
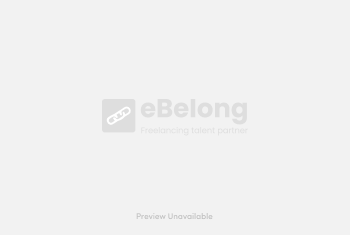
Looking for a role at a prestigious orga...
An ML Engineer or Machine Learning Engineer is a skilled professional who specializes in designing, developing, and deploying machine learning (ML) systems. They work at the intersection of software engineering and machine learning, bridging the gap between technical concepts and practical applications.
Core Responsibilities of an ML Engineer:
-
Machine Learning System Design: Architecting and designing ML systems, including data ingestion, feature engineering, model selection, and training pipelines.
-
Machine Learning Model Development: Implementing and training machine learning models using various algorithms and techniques, such as supervised learning, unsupervised learning, and reinforcement learning.
-
Machine Learning Experimentation and Evaluation: Conducting experiments to evaluate the performance of ML models, identifying areas for improvement, and optimizing model parameters.
-
Machine Learning System Deployment and Maintenance: Deploying and integrating ML models into production environments, monitoring their performance, and making adjustments as needed.
-
Machine Learning Infrastructure and Tools: Managing and optimizing ML infrastructure, including cloud platforms, data storage, and computational resources.
-
Collaboration with Data Scientists and Stakeholders: Collaborating with data scientists, domain experts, and stakeholders to understand business requirements and implement effective ML solutions.
Essential Skills and Qualifications for an ML Engineer:
-
Strong Programming Expertise: Proficiency in programming languages like Python, R, or Java for data manipulation, model implementation, and system development.
-
Machine Learning Fundamentals: In-depth understanding of machine learning concepts, algorithms, and techniques, including supervised learning, unsupervised learning, and deep learning.
-
Data Engineering Skills: Ability to handle large datasets, perform data cleaning and preparation, and extract relevant features for machine learning models.
-
Software Engineering Skills: Familiarity with software engineering principles, design patterns, and best practices for building scalable and maintainable systems.
-
Mathematical and Statistical Background: Understanding of mathematics, statistics, and linear algebra for comprehending machine learning algorithms and modeling.
-
Problem-Solving and Analytical Skills: Ability to identify and solve complex problems using machine learning techniques, analyze data, and draw meaningful conclusions.
-
Communication Skills: Ability to communicate technical concepts clearly to both technical and non-technical audiences, explaining the rationale behind ML solutions and their impact on business objectives.
-
Continuous Learning and Adaptability: Ability to stay abreast of emerging technologies and advancements in the field of machine learning, adapting to new tools and techniques as needed.